Dynamic Programming in 5 easy steps - Examples - Text Justification
Dynamic Programming is considered as one of the hardest methods to master, with few examples on the internet. Let’s contribute a little with this post series. Today I will cover the first problem - text justification. Credits: MIT lectures.
Algorithms and Data Structures it’s a broad subject, but for me personally I found the Dynamic Programming as the abandoned and unused method. It’s also considered as one of the hardest methods to master, with few examples on the internet. The problem for today is from MIT lectures and I highly recommend well explained videos along with notes from course 6.006: Introduction to algorithms - fall 2011.
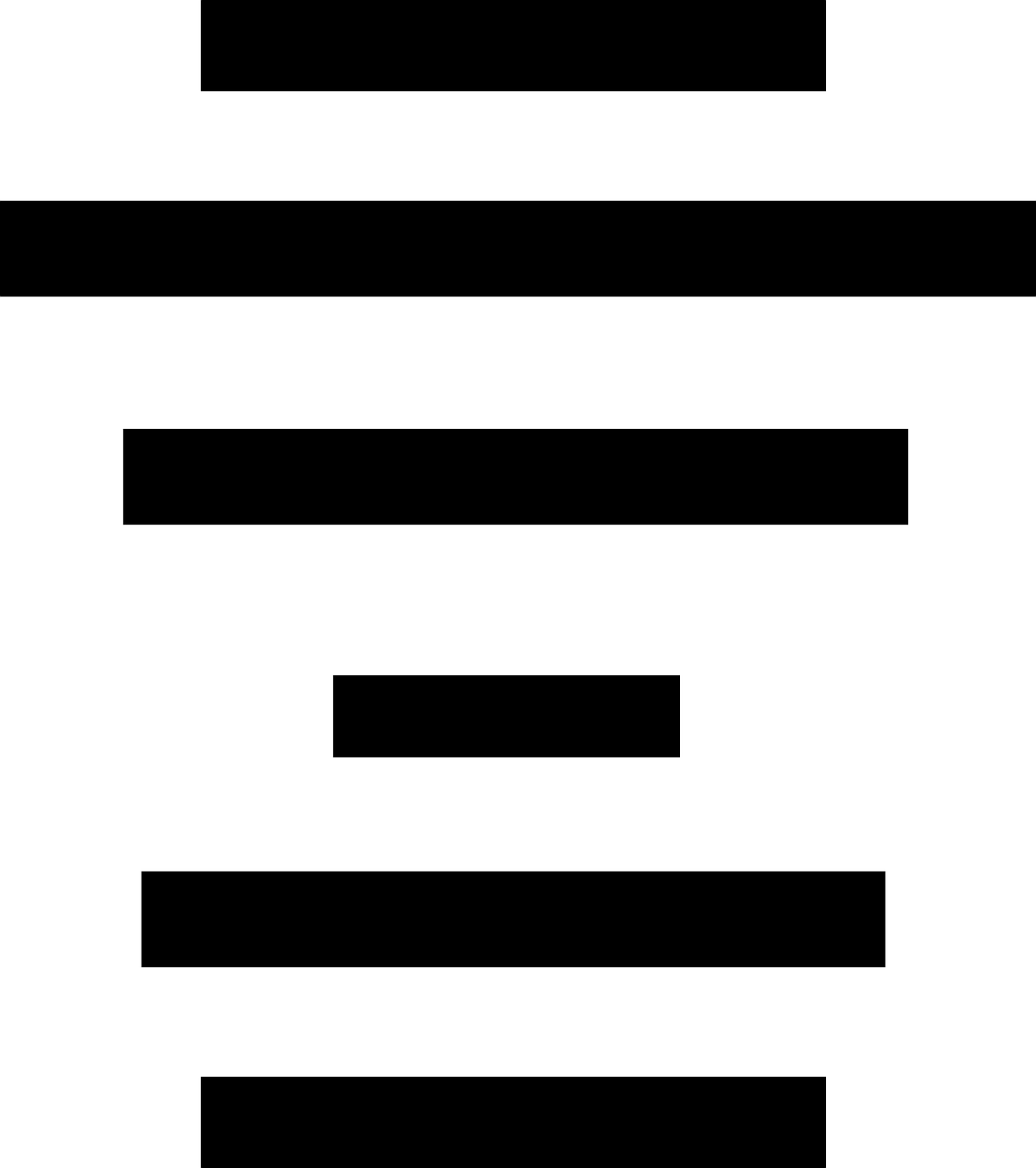
Text justification
Given a text, split it into multiple lines so it is “nicely” distributed between each line.What does it mean? Take a look at the example:
We can clearly see that eager approach won't produce good results in all cases.
Mathematically, given a badness(i, j) function for line of words[i : j], we seek for such a splitting that the sum of all badness is minimum.
Let's assume the badness function is predefined as follows:
∞ if total length > page width, else (page width − total length)3So in the example above, the bad splitting would have:
- page width = 16
- first line length = 4+4+4+2 = 16, badness of the first line = 0
- second line length = 4, badness = (16-4)3=1728
- third line length = 16, badness = 0
- badness sum = 1728
The good splitting would have:
- first line length = 4+4+1 = 9, badness of the first line = (16-9)3=343
- second line length = 9, badness = (16-9)3=343
- third line length = 16, badness = 8
- badness sum = 694
The second splitting has smaller total badness sum, thus it's a better splitting.
Dynamic Programming in 5 easy steps
On the MIT course, there is presented an approach to DP in 5 easy steps. Those are:
- define subproblems, count number of subproblems
- guess (part of solution), count number of choices
- relate subproblem solutions, compute time per subproblem
- recurse + memoize OR build DP table bottom-up check subproblems acyclic/topological order, time = time per subproblem · number of subproblems
- solve original problem: = a subproblem OR by combining subproblem solutions ⇒ extra time
Well, the steps don't have to be executed always in order. Let's try to figure out the second step. We know where our line begins, it's on ith position. Where is the end of the line? We don't know. So let's check all possibilities, that's from ith onward and take the smallest badness. So now we know what's our subproblems in first step are - suffixes of word[i:]. That's pretty much the algorithm. The third step will look like:
DP(i)=for j in i+1...n+1 takeThe great thing is about DP that DP(j) considered to be free (because we compute the subproblem once and memorize the answer). Therefore the total time needed by this algorithm is:
min from (DP(j) + badness(i, j))
time = time per subproblem · number of subproblems, soThe fourth point is about proving that this really works and the time needed is finite. We compute the answer from the end of the words, down to word number 0. The recursive DP function always accesses greater indexes, therefore time needed is finite. Lastly, we have to find our answer. Here, obviously it's DP[0]. Easy? Yup, let's code it!
time = O(n) · O(n)
time = O(n^2)
The code
1 |
|
If we don't care about justifying the last line, we can easily modify the badness function to return no cost for the last line:
1 |
|